Time Perception
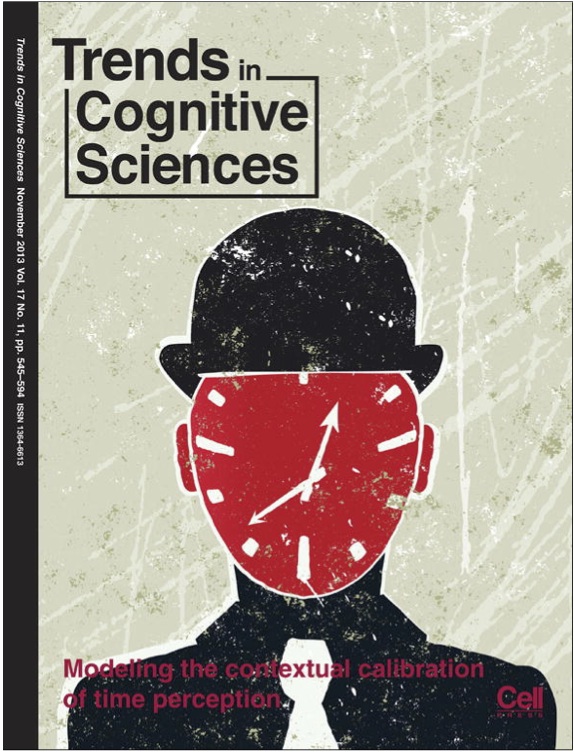
The sense of time, unlike other senses, is not produced by a specific sensory organ. Rather, all events that stimulate the brain, regardless of sensory modality, convey temporal cues. Due to heterogeneous processing of sensory events, subjective time may differ signficantly for a given duration across modality. For example, an auditory event is often perceived longer than a visual event of the same phyiscal inerval. Subjective time is also susceptible to temporal context, voluntary actions, attention, arousal and emotional states. In this research topic, we focus on mechanisms underlying temporal process and multisensory integration in time perception using behavioral investigation and Bayesian modeling.
Related Research projects
Dynamic priors and contextual calibration (DFG 166/3-1, 3-2)
The projects aim to uncover the mechanism underlying dynamic contextual calibration in multimodal environments, and to develop a general Bayesian framework describing the prior updating mechanisms in contextual calibration. In particular, we plan to focus on central tendency effects in magnitude estimation. The central tendency effect (also known as the range or regression effect), which has been well documented in the literature (e.g., Helson, 1963; for a review, see Shi, Church, & Meck, 2013), refers to a bias engendered by prior knowledge of the sampled distribution of stimuli presented. Although central tendency effects have been found in various types of sensory estimation, there is at present no consensus on what ranges of statistical information are actually involved in contextual calibration. This issue becomes particularly prominent for multisensory stimuli, given that multiple statistics (priors) are available which are not always consistent with each other. Here, we plan to establish a general computational framework to examine whether the brain uses multiple modality-specific priors or an amodal prior in contextual calibration, and how the brain resolves inconsistencies among priors, as well as how action priors are taken into consideration in magnitude estimation. In addition, we will further develop trial-wise computational Bayesian models (e.g., Kalman filter, particle filters, hierarchical models) to achieve a better prediction of dynamic prior updating and contextual calibration.
Selected publications:
- Ren, Y., Allenmark, F., Müller, H. J., & Shi, Z. (2021). Variation in the “coefficient of variation”: Rethinking the violation of the scalar property in time-duration judgments. Acta Psychologica, 214, 103263. https://doi.org/10.1016/j.actpsy.2021.103263
- Ren, Y., Allenmark, F., Müller, H. J., & Shi, Z. (2020). Logarithmic encoding of ensemble time intervals. Scientific Reports, 10(1), 18174. https://doi.org/10.1038/s41598-020-75191-6
- Zhu, X., Baykan, C., Müller, H. J., & Shi, Z. (2020). Temporal bisection is influenced by ensemble statistics of the stimulus set. Attention, Perception & Psychophysics. https://doi.org/10.3758/s13414-020-02202-z
- Chen, L., Zhou, X., Müller, H. J., & Shi, Z. (2018). What you see depends on what you hear: Temporal averaging and crossmodal integration. Journal of Experimental Psychology. General, 147(12), 1851–1864. https://doi.org/10.1037/xge0000487
- Jia, L., & Shi, Z.* (2017). Duration compression induced by visual and phonological repetition of Chinese characters. Attention, Perception, & Psychophysics, 79(7), p. 2224-2232. http://doi.org/10.3758/s13414-017-1360-3
- Gu, B., Jurkowski A.J., Shi,Z., Meck W.H. (2016) Bayesian optimization of interval timing and biases in temporal memory as a function of temporal context, feedback, and dopamine levels in young, aged and Parkinson’s Disease patients, Timeing & Timing Perception, p. 1-27, doi:10.1163/22134468-00002072
- Shi, Z., & Burr, D. (2016). Predictive coding of multisensory timing, Current Opinion in Behavioral Sciences, http://dx.doi.org/10.1016/j.cobeha.2016.02.014
- Shi, Z., Church, R. M., Meck, W. H. (2013), Bayesian optimization of time perception, Trends in Cognitive Sciences, 17(11), 556-564. DOI:10.1016/j.tics.2013.09.009
- Shi, Z., Ganzenmüller, S., & Müller, H. J. (2013). Reducing Bias in Auditory Duration Reproduction by Integrating the Reproduced Signal. (W. H. Meck, Ed.)PLoS ONE, 8(4), e62065. doi:10.1371/journal.pone.0062065
- Shi, Z., Jia, L., Müller, H. J. (2012), Modulation of tactile duration judgments by emotional pictures, Front. Integr. Neurosci. 6:24. doi: 10.3389/fnint.2012.00024
- Ganzenmüller, S., Shi, Z., & Müller, H. J. (2012). Duration reproduction with sensory feedback delay: differential involvement of perception and action time. Frontiers in Integrative Neuroscience, 6(October), 1–11. doi:10.3389/fnint.2012.00095
Contextual learning and modulation
Our external environment is often structured and relative stable. Surrounding contexts can be useful for guiding our attention and selection. However, changes of background context can also bias our perception. In this research topic, we aim to investigate the following aspects of contextual learning and modulation in our perception:
- Contextual cueing in visual/tactile search
- Probability cueing in guided search
- Serial dependence and contextual modulation in magnitude estimation
Related Research Projects
DFG Project: Mechanisms of spatial context learning through touch and vision (together with Thomas Geyer)
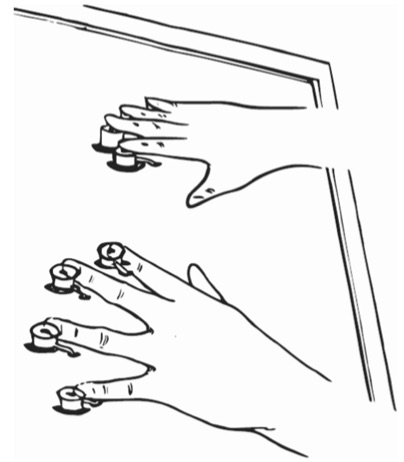
In everyday scenes, searched-for targets do not appear in isolation, but are embedded within configurations of non-target or distractor items. If the position of the target relative to the distractors is invariant, such spatial contingencies are learned and come to guide environmental scanning (“contextual cueing” effect; (Chun & Jiang, 1998; Geyer, Shi, & Müller, 2010). Importantly, such context learning is not limited to the visual modality: using a novel tactile search task, we recently showed that repeated tactile contexts aid tactile search (Assumpção, Shi, Zang, Müller, & Geyer, 2015, 2017). However, the guidance of search by context memory has almost entirely been investigated in the individual modalities of vision and touch. Thus, relatively little is known about crossmodal plasticity in spatial context learning. The present proposal will employ behavioural and neuroscience methods, in addition to mathematical modelling, to investigate context learning in unimodal search tasks (consisting of only visual or tactile search items), and multimodal and crossmodal search tasks (consisting of both visual and tactile search items). In doing so, any specific learning mechanisms (representations) commonly referred to as crossmodal plasticity should be revealed. The three leading research questions concern (1) the factors that may eventually give rise to crossmodal context memory (an example question is whether the development of crossmodal spatial context memory requires practice on a multimodal search task; (2) how context memory acquired in uni- or multimodal search tasks is characterized in ERP waveforms (and whether there are differences in electrophysiological components between these tasks); and (3), how the beneficial effects of context memory on reaction time performance in uni- and multimodal search can be quantified using stochastic Bayesian models.
DFG Project: The construction of attentional templates in cross-modal pop-out search (Co-PI with Thomas Töllner, Hermann Müller)
How do we select information that is relevant to achieve our current action goals from the plethora of information, which streams into our brain continuously via our sensory organs?One prominent notion according to which selection of relevant items may be accomplished is the engagement of “attentional templates”. Following this idea, we may set up an internal mental image of the desired object in working memory (WM), which represents the target’s feature values—including, for example, it’s color, shape, or size. This WM representation may be then linked – via recurrent feedback connections – to early sensory analyzer units, and boost the output of those neuronal units that code the respective target features. As a result, objects containing one or several target features will have a selection advantage relative to objects that contain currently irrelevant features. The second phase of project B1 is designed to explore whether this theoretical framework is limited to visual targets, or, alternatively, can also explain target selection in other sensory modalities (e.g., touch). Re-using our previously established multisensory pop-out search paradigm, we will combine behavioural (reaction times, accuracy) and electrophysiological measures (event-related lateralizations, oscillations) with computational modelling (priors). In particular, we aim at examining whether one-and-the-same attentional template can integrate information from two different sensory modalities. Or, alternatively, whether search for cross-modally defined targets requires the setting up and maintenance of two separate, uni-sensory attentional templates. The outcome of the proposed research programme will advance our understanding of the hierarchical processing architecture that underlies cross-modal search.
Selected Publications
- Chen, S., Shi, Z., Müller, H. J., & Geyer, T. (2021). Multisensory visuo-tactile context learning enhances the guidance of unisensory visual search. Scientific Reports, 11(1), 9439. https://doi.org/10.1038/s41598-021-88946-6
- Zhang, B., Weidner, R., Allenmark, F., Bertleff, S., Fink, G. R., Shi, Z., & Müller, H. J. (2021). Statistical learning of frequent distractor locations in visual search involves regional signal suppression in early visual cortex. In bioRxiv (p. 2021.04.16.440127). https://doi.org/10.1101/2021.04.16.440127
- Allenmark, F., Shi, Z., Pistorius, R. L., Theisinger, L. A., Koutsouleris, N., Falkai, P., Müller, H. J., & Falter-Wagner, C. M. (2020). Acquisition and Use of “Priors” in Autism: Typical in Deciding Where to Look, Atypical in Deciding What Is There. Journal of Autism and Developmental Disorders. https://doi.org/10.1007/s10803-020-04828-2
- Chen, S., Shi, Z., Müller, H. J., & Geyer, T. (2021). When visual distractors predict tactile search: The temporal profile of cross-modal spatial learning. Journal of Experimental Psychology. Learning, Memory, and Cognition. https://doi.org/10.1037/xlm0000993
- Chen, S., Shi, Z., Zang, X., Zhu, X., Assumpção, L., Müller, H. J., & Geyer, T. (2019). Crossmodal learning of target-context associations: When would tactile context predict visual search? Attention, Perception & Psychophysics. https://doi.org/10.3758/s13414-019-01907-0
- Allenmark, F., Zhang, B., Liesefeld, H. R., Shi, Z., & Müller, H. J. (2019). Probability cueing of singleton-distractor regions in visual search: the locus of spatial distractor suppression is determined by colour swapping. Visual Cognition, 1–19. https://doi.org/10.1080/13506285.2019.1666953
- Shi, Z., Allenmark, F., Zhu, X., Elliott, M. A., & Müller, H. J. (2019). To quit or not to quit in dynamic search. Attention, Perception & Psychophysics. https://doi.org/10.3758/s13414-019-01857-7
- Zhang, B., Allenmark, F., Liesefeld, H. R., Shi, Z., & Müller, H. J. (2019). Probability cueing of singleton-distractor locations in visual search: Priority-map- versus dimension-based inhibition? Journal of Experimental Psychology. Human Perception and Performance, 45(9), 1146–1163. https://doi.org/10.1037/xhp0000652
- Assumpcao, L., Shi, Z., Zang, X., Müller, H. J., Geyer, T. (2018). Contextual cueing of tactile search is supported by an anatomical reference frame. J Exp Psychol Hum Percept Perform, 44(4): 566-577. doi: 10.1037/xhp0000478.
- Zang, X., Shi, Z., Müller, H. J., & Conci, M. (2017). Contextual cueing in 3D visual search depends on representations in planar-, not depth-defined space. Journal of Vision, 17(5), 17. http://doi.org/10.1167/17.5.17
- Shi, Z., Zang, X., & Geyer, T. (2017). What fixations reveal about oculomotor scanning behavior in visual search. Behavioral and Brain Sciences, 40, e155. http://doi.org/10.1017/S0140525X1600025X
- Zang, X., Geyer, T., Assumpção, L., Müller, H.J., Shi, Z. (2016) From Foreground to Background: How Task-Neutral Context Influences Contextual Cueing of Visual Search, Frontiers in Psychology 7(June), p. 1-14, url, doi:10.3389/fpsyg.2016.00852
- Assumpção, L., Shi, Z., Zang, X., Müller, H. J., & Geyer, T. (2015). Contextual cueing: implicit memory of tactile context facilitates tactile search. Attention, Perception, & Psychophysics. doi:10.3758/s13414-015-0848-y
- Zang, X., Jia, L., Müller, H. J., Shi, Z. (2014), Invariant spatial context is learned but not retrieved in gaze-contingent tunnel-view search, Journal of Experimental Psychology: Learning, Memory, and Cognition. http://www.ncbi.nlm.nih.gov/pubmed/25329087.
Bayesian Inference
In this research topic we apply Bayesian modeling on various types of contextual biases (e.g., serial dependence, central tendency effect), and on search performance by combining Bayesian inference and drift-diffusion model.
Related Research Projects
DFG Project: Bayesian modeling of hierarchical prior and its updating mechanisms (together with Stefan Glasauer)
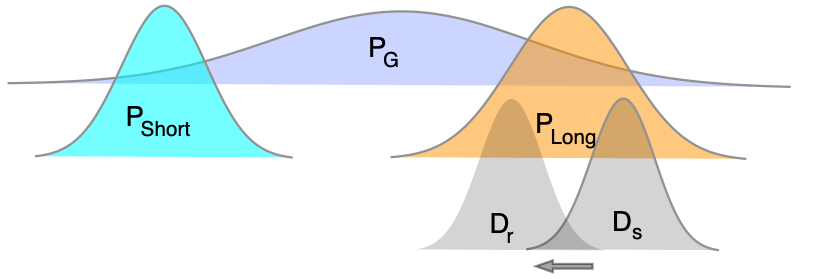
Uncertainty is ubiquitous in all perceptual processes and decision-making. To cope with uncertainty, our brain relies on prediction and interpretation of sensory inputs, which are inseparable characteristics of internal models and prior knowledge. However, incorporating prior information could also engender systematic biases, such as the central-tendency effect that manifests in overestimation for short magnitudes and underestimation for long magnitudes in a set of stimuli. Recently, these systematic biases have been interpreted in a Bayesian framework. Although Bayesian integration of prior information has been found across a wide range of stimulus settings, to date there is very little consensus on what prior structures precisely are actually involved in contextual modulation, and how cognitive load and sequential dependence influence internal prior(s) and sensory estimates. The overarching goals of the current project are to identify the hierarchical structure of the internal prior, to uncover how sequential dependence and working memory load influence the prior updating, and to develop trial-wise Bayesian updating models to better account for empirical findings. Specifically, we plan to use the central-tendency effect that arises in production- reproduction tasks (in both the temporal and spatial domains) as a testbed for establishing and testing a general Bayesian computational framework.
DFG Project: Modeling predictive weighting systems in visual system (Together with Hermann J. Müller)
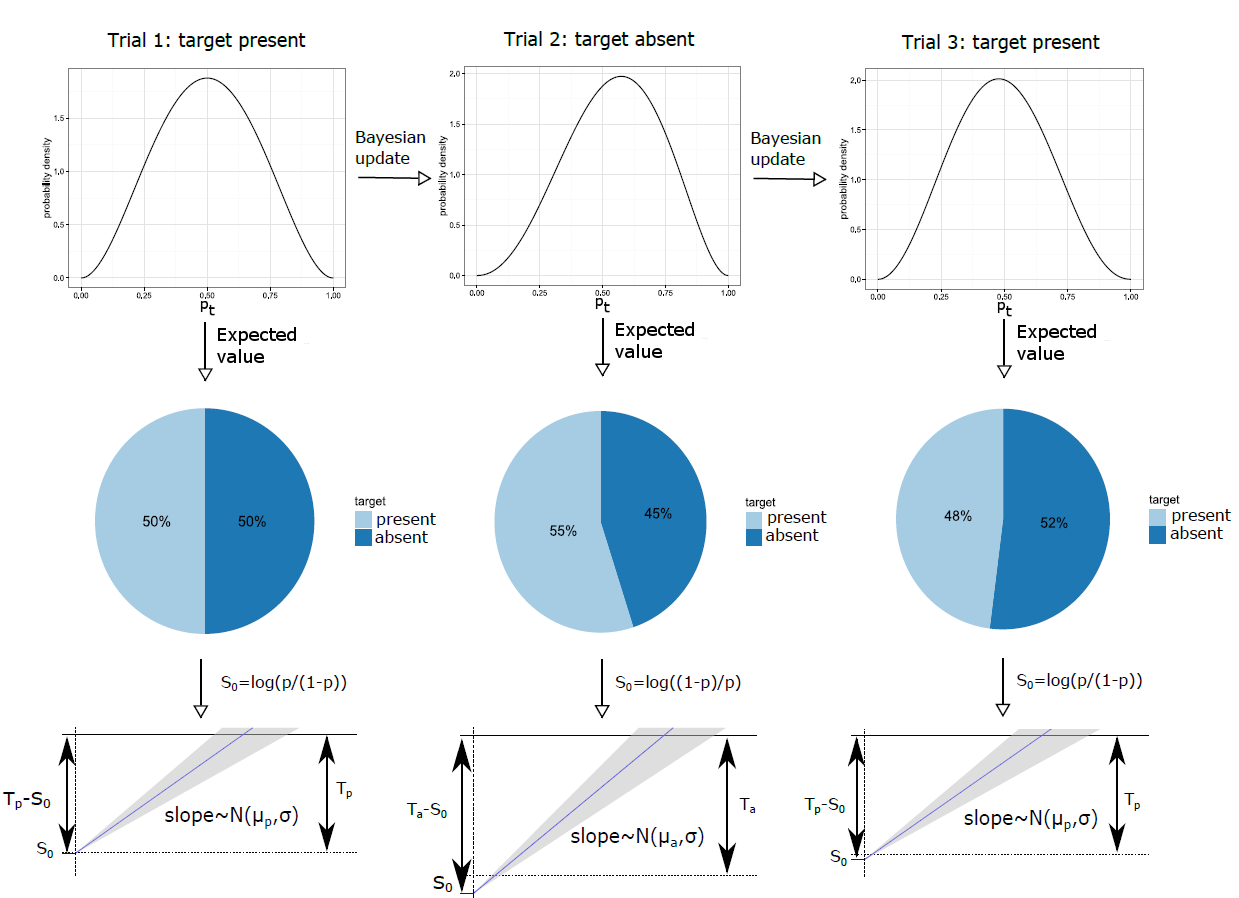
The aim of the project is to develop a principled, quantitative framework describing dynamic weighting processes in visual search. In particular, we plan to model different types of inter-trial effects, foremost: the effects of repetitions vs. switches of the target-defining (features and) dimensions in singleton-target search scenarios. These effects have been well characterized qualitatively over the past decades and led to the notion of ‘dimension weighting’ (which has now become an accepted component of saliency-based accounts of visual search, including J. M. Wolfe’s Guided Search model). Although inter-trial dynamics is sometimes still seen as only a ‘minor’ source of search RT variation, it actually accounts for a large portion of response time (RT) variability even in the most simple, supposedly purely stimulus-driven singleton feature ‘pop-out’ search (e.g., Found & Müller, 1996), and an even larger portion in singleton conjunction search (e.g., Weidner et al., 2002) – and is arguably more influential than top-down, template-based) influences on search performance (e.g., Kristjánsson et al., 2002). However, while clearly important and well characterized, we are still lacking a principled, computational account of the dynamics of weighting. Here, we propose to develop such an account by combining a new, Bayesian-type perceptual decision and weight updating model with a generative model of RT distributions. In more detail, as singleton feature search is driven largely by target saliency, we propose to use the LATER (‘Linear Approach to Threshold with Ergodic Rate’) model for modeling the RT distributions. On top of this, we will develop a perceptual model accounting for the influences of prior knowledge on target detection, (feature- and dimension-based) inter-trial effects, as well as dimension-weighting mechanisms. In addition, we will revisit the notion of coactive processing of redundant pop-out signals and examine its relation to inter-trial effects. In a second project phase, we plan to generalize our perceptual-response framework to a singleton search paradigm not limited to the pop-out search, as well as looking for brain correlates of the weighting dynamics.
Related Publications
- Ren, Y., Allenmark, F., Müller, H. J., & Shi, Z. (2021). Variation in the “coefficient of variation”: Rethinking the violation of the scalar property in time-duration judgments. Acta Psychologica, 214, 103263. https://doi.org/10.1016/j.actpsy.2021.103263
- Zhu, X., Baykan, C., Müller, H. J., & Shi, Z. (2020). Temporal bisection is influenced by ensemble statistics of the stimulus set. Attention, Perception & Psychophysics. https://doi.org/10.3758/s13414-020-02202-z
- Chen, L., Zhou, X., Müller, H. J., & Shi, Z. (2018). What you see depends on what you hear: Temporal averaging and crossmodal integration. Journal of Experimental Psychology. General, 147(12), 1851–1864. https://doi.org/10.1037/xge0000487
- Glasauer, S., Shi, Z. (2018) 150 years of research on Vierordt’s law – Fechner’s fault? [BioRxiv] doi: 10.1101/ 450726
- Chen, L., Zhou, X., Müller, H. J., Shi, Z.* (2018) What you see depends on what you hear:
temporal averaging and crossmodal integration, JEP: General, doi:10.1037/xge0000487 [BioRxiv] [Experimental Data and Source Code] - Allenmark, F., Shi, Z., Müller, H. J. (2018) Inter-trial effects in visual search: Factorial comparison of Bayesian updating models, PLOS Computational Biology, e1006328, doi:10.1371/journal.pcbi.1006328 [BioRxiv] [Experimental Data and Source Code]
- Gu, B., Jurkowski A.J., Shi,Z., Meck W.H. (2016) Bayesian optimization of interval timing and biases in temporal memory as a function of temporal context, feedback, and dopamine levels in young, aged and Parkinson’s Disease patients, Timeing & Timing Perception, p. 1-27, doi:10.1163/22134468-00002072
- Shi, Z., & Burr, D. (2016). Predictive coding of multisensory timing, Current Opinion in Behavioral Sciences, http://dx.doi.org/10.1016/j.cobeha.2016.02.014
- Rank, M., Shi, Z., Müller, H. J., & Hirche, S. (2016). Predictive Communication Quality Control in Haptic Teleoperation with Time Delay and Packet Loss. IEEE Transactions on Human-Machine Systems, doi: 10.1109/THMS.2016.2519608.