In this research topic we apply Bayesian modeling on various types of contextual biases (e.g., serial dependence, central tendency effect), and on search performance by combining Bayesian inference and drift-diffusion model.
Related Research Projects
DFG Project: Bayesian modeling of hierarchical prior and its updating mechanisms (together with Stefan Glasauer)
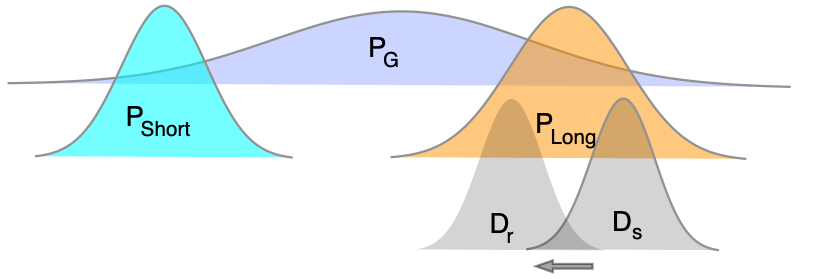
Uncertainty is ubiquitous in all perceptual processes and decision-making. To cope with uncertainty, our brain relies on prediction and interpretation of sensory inputs, which are inseparable characteristics of internal models and prior knowledge. However, incorporating prior information could also engender systematic biases, such as the central-tendency effect that manifests in overestimation for short magnitudes and underestimation for long magnitudes in a set of stimuli. Recently, these systematic biases have been interpreted in a Bayesian framework. Although Bayesian integration of prior information has been found across a wide range of stimulus settings, to date there is very little consensus on what prior structures precisely are actually involved in contextual modulation, and how cognitive load and sequential dependence influence internal prior(s) and sensory estimates. The overarching goals of the current project are to identify the hierarchical structure of the internal prior, to uncover how sequential dependence and working memory load influence the prior updating, and to develop trial-wise Bayesian updating models to better account for empirical findings. Specifically, we plan to use the central-tendency effect that arises in production- reproduction tasks (in both the temporal and spatial domains) as a testbed for establishing and testing a general Bayesian computational framework.
DFG Project: Modeling predictive weighting systems in visual system (Together with Hermann J. Müller)
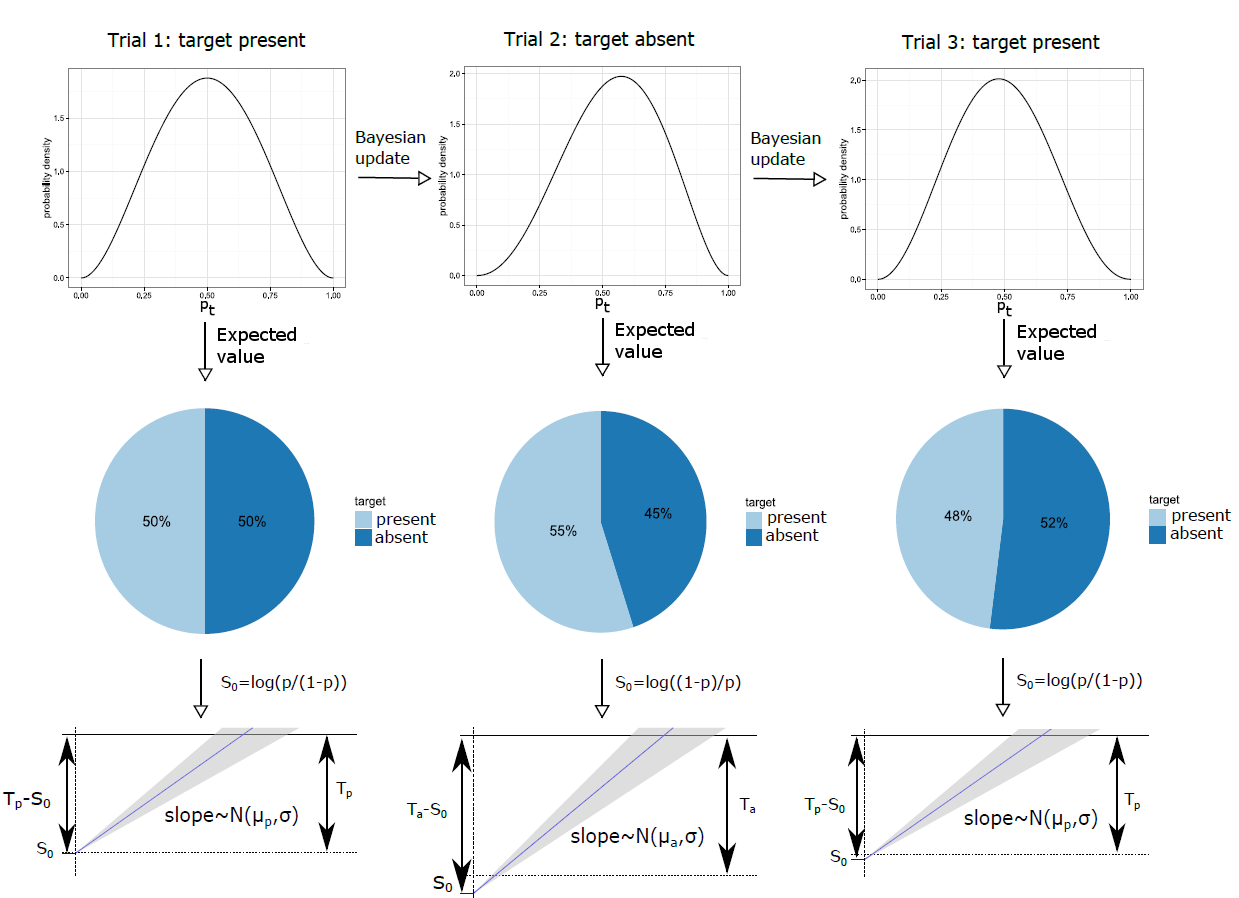
The aim of the project is to develop a principled, quantitative framework describing dynamic weighting processes in visual search. In particular, we plan to model different types of inter-trial effects, foremost: the effects of repetitions vs. switches of the target-defining (features and) dimensions in singleton-target search scenarios. These effects have been well characterized qualitatively over the past decades and led to the notion of ‘dimension weighting’ (which has now become an accepted component of saliency-based accounts of visual search, including J. M. Wolfe’s Guided Search model). Although inter-trial dynamics is sometimes still seen as only a ‘minor’ source of search RT variation, it actually accounts for a large portion of response time (RT) variability even in the most simple, supposedly purely stimulus-driven singleton feature ‘pop-out’ search (e.g., Found & Müller, 1996), and an even larger portion in singleton conjunction search (e.g., Weidner et al., 2002) – and is arguably more influential than top-down, template-based) influences on search performance (e.g., Kristjánsson et al., 2002). However, while clearly important and well characterized, we are still lacking a principled, computational account of the dynamics of weighting. Here, we propose to develop such an account by combining a new, Bayesian-type perceptual decision and weight updating model with a generative model of RT distributions. In more detail, as singleton feature search is driven largely by target saliency, we propose to use the LATER (‘Linear Approach to Threshold with Ergodic Rate’) model for modeling the RT distributions. On top of this, we will develop a perceptual model accounting for the influences of prior knowledge on target detection, (feature- and dimension-based) inter-trial effects, as well as dimension-weighting mechanisms. In addition, we will revisit the notion of coactive processing of redundant pop-out signals and examine its relation to inter-trial effects. In a second project phase, we plan to generalize our perceptual-response framework to a singleton search paradigm not limited to the pop-out search, as well as looking for brain correlates of the weighting dynamics.
Related Publications
- Ren, Y., Allenmark, F., Müller, H. J., & Shi, Z. (2021). Variation in the “coefficient of variation”: Rethinking the violation of the scalar property in time-duration judgments. Acta Psychologica, 214, 103263. https://doi.org/10.1016/j.actpsy.2021.103263
- Zhu, X., Baykan, C., Müller, H. J., & Shi, Z. (2020). Temporal bisection is influenced by ensemble statistics of the stimulus set. Attention, Perception & Psychophysics. https://doi.org/10.3758/s13414-020-02202-z
- Chen, L., Zhou, X., Müller, H. J., & Shi, Z. (2018). What you see depends on what you hear: Temporal averaging and crossmodal integration. Journal of Experimental Psychology. General, 147(12), 1851–1864. https://doi.org/10.1037/xge0000487
- Glasauer, S., Shi, Z. (2018) 150 years of research on Vierordt’s law – Fechner’s fault? [BioRxiv] doi: 10.1101/ 450726
- Chen, L., Zhou, X., Müller, H. J., Shi, Z.* (2018) What you see depends on what you hear:
temporal averaging and crossmodal integration, JEP: General, doi:10.1037/xge0000487 [BioRxiv] [Experimental Data and Source Code] - Allenmark, F., Shi, Z., Müller, H. J. (2018) Inter-trial effects in visual search: Factorial comparison of Bayesian updating models, PLOS Computational Biology, e1006328, doi:10.1371/journal.pcbi.1006328 [BioRxiv] [Experimental Data and Source Code]
- Gu, B., Jurkowski A.J., Shi,Z., Meck W.H. (2016) Bayesian optimization of interval timing and biases in temporal memory as a function of temporal context, feedback, and dopamine levels in young, aged and Parkinson’s Disease patients, Timeing & Timing Perception, p. 1-27, doi:10.1163/22134468-00002072
- Shi, Z., & Burr, D. (2016). Predictive coding of multisensory timing, Current Opinion in Behavioral Sciences, http://dx.doi.org/10.1016/j.cobeha.2016.02.014
- Rank, M., Shi, Z., Müller, H. J., & Hirche, S. (2016). Predictive Communication Quality Control in Haptic Teleoperation with Time Delay and Packet Loss. IEEE Transactions on Human-Machine Systems, doi: 10.1109/THMS.2016.2519608.